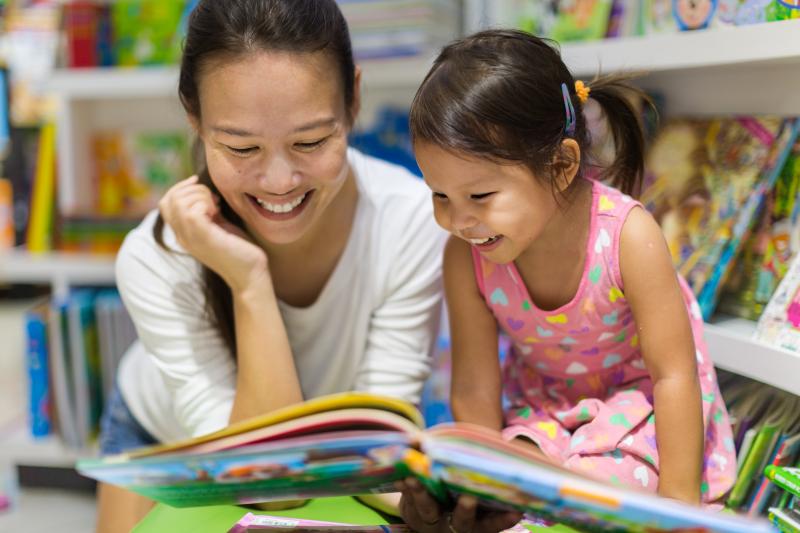
Embedded in electronic health record (EHR) data are the stories of thousands of late-talking children and how their communication abilities develop over time, what co-occurring conditions they may have, and which services they may be accessing.
Benjamin Goldstein, PhD, director of data science at Duke AI Health, and Lauren Franz, MBChB, MPH, interim director of Duke Center for Autism and Brain Development, believe these “stories” could help reveal developmental pathways for late-talking children. However, EHRs contain far too much information for a research team to read and analyze.
That’s why their new study is employing large language models, a type of artificial intelligence that enables machines to understand human language, to automatically recognize and interpret clinical notes related to late talking.
By 24 months of age, most children say at least 50 words and use some two-word combinations. But one in five children in the United States don’t reach this developmental milestone at the expected time. These children may receive a diagnosis of late language emergence, or “late talking.” Parents and caregivers may wonder about long-term outcomes for their child’s communication, and pediatricians face the question of which late-talking children will need specialized assessment and intervention to support language development. Some children with signs of delayed language development are not diagnosed with late talking at their well-child visit, and may miss out on early therapies and supports. A better understanding of specific developmental pathways associated with late talking would improve the clinician’s ability to identify and connect the right child with the right early intervention and supports.
Duke and North Carolina Central University (NCCU) researchers are looking to uncover stories embedded in EHR that can help us understand their development. In 2024, the National Institutes of Health created the Tackling Acquisition of Language in Kids (TALK) Initiative to support research activities that contribute to building this knowledge base, a congressionally-mandated area of research interest and support.
With the support of two grants from the TALK initiative, Goldstein and Franz and their team are exploring how EHR data can help us understand developmental pathways for late-talking children. Another focus for the project is the health equity dimensions of late talking, including differences across gender, language, race, ethnicity, insurance type and socioeconomic status.
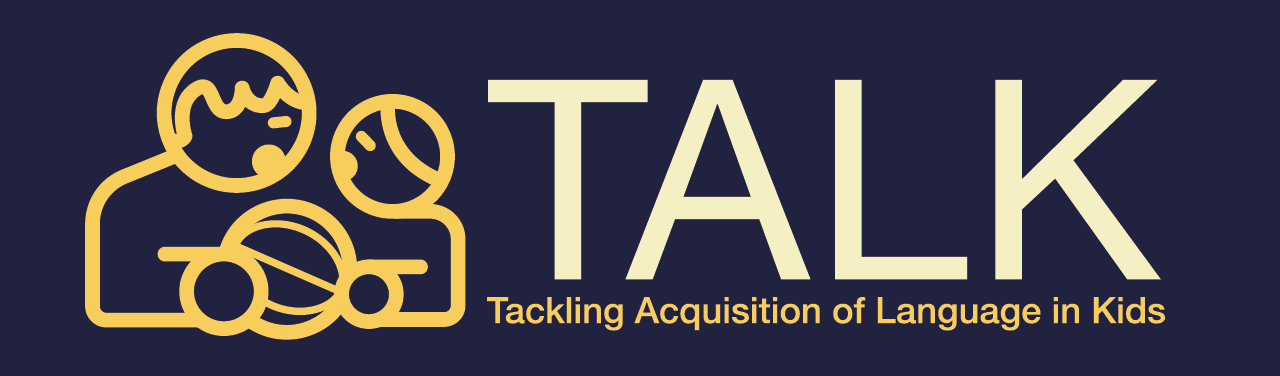
Their co-investigators include Danai Fannin, PhD, a speech-language pathology specialist and associate professor of communications sciences and disorders at NCCU; Duke AI Health data science fellowship director Matthew Engelhard, MD, PhD; and pediatrician and child psychiatrist Gary Maslow, MD, MPH, medical director of the Duke Center for Autism and Brain Development.
To date, late talking research has mostly relied on data from small studies with relatively few participants who are not fully representative of all the children growing up in America today. Real-world EHR data allows the team to consider a larger, more representative group of children over a longer period of time.
Previous studies on late talking have also relied on International Classification of Diseases (ICD) coding to identify late-talking children. However, late talking may be under-coded, with clinicians entering no formal diagnostic code in the medical record when concerns are raised by caregivers about their child’s language development. Factors related to race, sex, home language, and insurance status also may impact whether late talking is coded in the medical record. The clinicians’ free-text notes from 18-24 month well-child visits may mention characteristics of late talking or describe caregiver concerns.
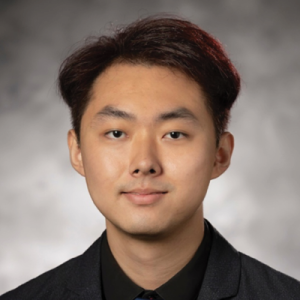
Jiang Shu, a graduate research assistant at Duke University working towards a Masters of Biostatistics, supports the team’s data analysis of the large language models. “The most interesting part is communicating with the clinicians throughout the project. Collaborating with the health care professionals enhanced my ability to communicate statistical findings into accessible language,” Shu said.
The goal of employing large language models is to identify patterns that sort the unique stories found in clinicians’ notes into a number of profiles with specific developmental trajectories. The team hopes that the collaboration between data analysts and healthcare professionals can help families understand what possibilities might come next for their child’s language development.