
You may be familiar with the saying, “If you’ve met one autistic person, you’ve met one autistic person”. In a clinical and research context, while we know a lot about how different autistic children present, this knowledge doesn’t always help us identify which groups of children may respond differently to specific supports and intervention approaches. Even experienced clinicians have a limited number of interventions to fit all these unique children.
Finding a Biomarker for Autism
Clinical trials for interventions need to identify metrics in advance to determine if the approach works. For example, a trial for a new blood pressure medication would take the participants’ blood pressure before and after using the medication to see if this measurement changed. This type of measurement is known as a biomarker. But there is no widely accepted autism biomarker. Most autism assessments are based on subjective information like caregivers’ reports in addition to clinicians’ observations.
Biomarkers for behaviors associated with autism could help categorize autistic children into groups that may respond differently to different intervention approaches, if needed, and match them with the most appropriate services and supports more quickly.
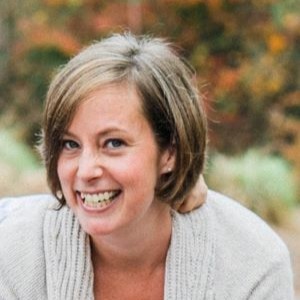
With that goal in mind, Kimberly Carpenter, PhD, and David Carlson, PhD, and their cross-disciplinary research team are conducting the Computer vision for Multi-Modal EEG Technology (COMET) study, supported by the Duke Autism Center of Excellence grant from the National Institute of Child Health and Human Development. Carpenter is an associate professor in Psychiatry and Behavioral Sciences and Carlson is an associate professor in Biostatistics & Bioinformatics, both at Duke.
We’re creating new and improved ways to measure autistic features using multiple types of data.
– Kimberly Carpenter, PhD
COMET is looking for the next generation of brain-based biomarkers using machine learning, a subfield of artificial intelligence that can identify patterns in large sets of data.
"What hasn’t been done is to combine brain-based biomarkers that have been studied for decades with tablet-based technology that we developed that can code behaviors using computer vision analysis. By combining these two things, we’re creating new and improved ways to measure autistic features using multiple types of data," Carpenter said.
COMET Measures Brain Activity Over Time
In the COMET study, children wear an electro-encephalogram (EEG) net, which measures brain activity while they watch short videos and play games on a tablet. The measurements are tracked over time as the child reaches behavioral milestones in order to learn how these changes are reflected in brain activity. The COMET team uses machine learning to analyze the data for differences or unique patterns in the synchronized behavior and brain activity of autistic and non-autistic children.
One potential biomarker that has been identified by other research groups is an EEG signal from the brain, known as N170. This EEG signal is associated with recognizing human faces. It been shown by the Autism Biomarkers Consortium for Clinical Trials (ABC-CT) to be measurably slower in some groups of autistic children.
The team is combining new technologies, including the computer vision models being developed at the Duke Center for Autism, to understand exactly what is happening in the brain while a child does different activities.
“The brain is incredibly complex, doing many things at the same time. In all of that data, what are the differences between neurotypical development and autism?” said Carlson. Using advanced techniques and statistical models, Carpenter, Carlson, and their team want to identify a reliable biomarker that can tell us what autism looks like in the brain.
Predictive Patterns
They measure the brain in a resting state, while the child watches a neutral video on a tablet, and also during social and non-social activities to see how the brain performs differently. All of this data, with every possible brain measurement, is entered into the computer program to analyze patterns.
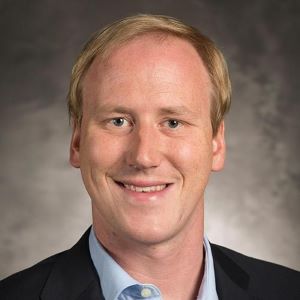
Rather than telling the computer how to calculate the data, Carlson explained, “We give the machine a lot of data and tell it to find patterns and differences.” The machine learning models are learning how to predict what formula will give the most reliable difference in the brain activity of neurodivergent and typically developing children.
The brain is incredibly complex, doing many things at the same time. In all of that data, what are the differences between neurotypical development and autism?
- David Carlson, PhD
“It finds things that are predictive, but finds a different way to predict it every time new data is put in. We’d expect that with a general pattern that describes how things are in reality, it would find a similar model every time,” said Carlson. He described how the team looks for the most consistent methods that the machine learning program uses across each simulation, and not necessarily its most confident prediction in any one run of the program.
Serving Families Through Research
To collect data for the computer to analyze, the COMET team provides diagnostic evaluations to participating families at no cost. By participating in research, some families are able to access care that they might not otherwise have access to. Elias Peters, a senior clinical research specialist on the COMET study, sees these families daily.
Scarcity, affordability, and other logistical challenges involved in diagnostic evaluations mean the needs of many children go unmet. The COMET study serves to alleviate this issue locally through our recruitment, and on a broader scale through the goals and potential applications of our research. It is exciting to be part of something that could benefit countless families in the future.
- Elias Peters, Sr. Clinical Research Specialist
Also a senior clinical research specialist, David Akinsooto serves as a point of contact for families, helps administer EEG sessions, and assists with data collection and analysis.
The COMET study has taught me a lot about how innovations in technology can help us understand more about autism, its associated behaviors, and the spectrum that it lies on. As the middle child of two autistic siblings, working on the COMET study has been a wonderful experience. I have always wanted to understand my siblings so that I could be the best advocate for them and the autistic community.
- David Akinsooto, Sr. Clinical Research Specialist
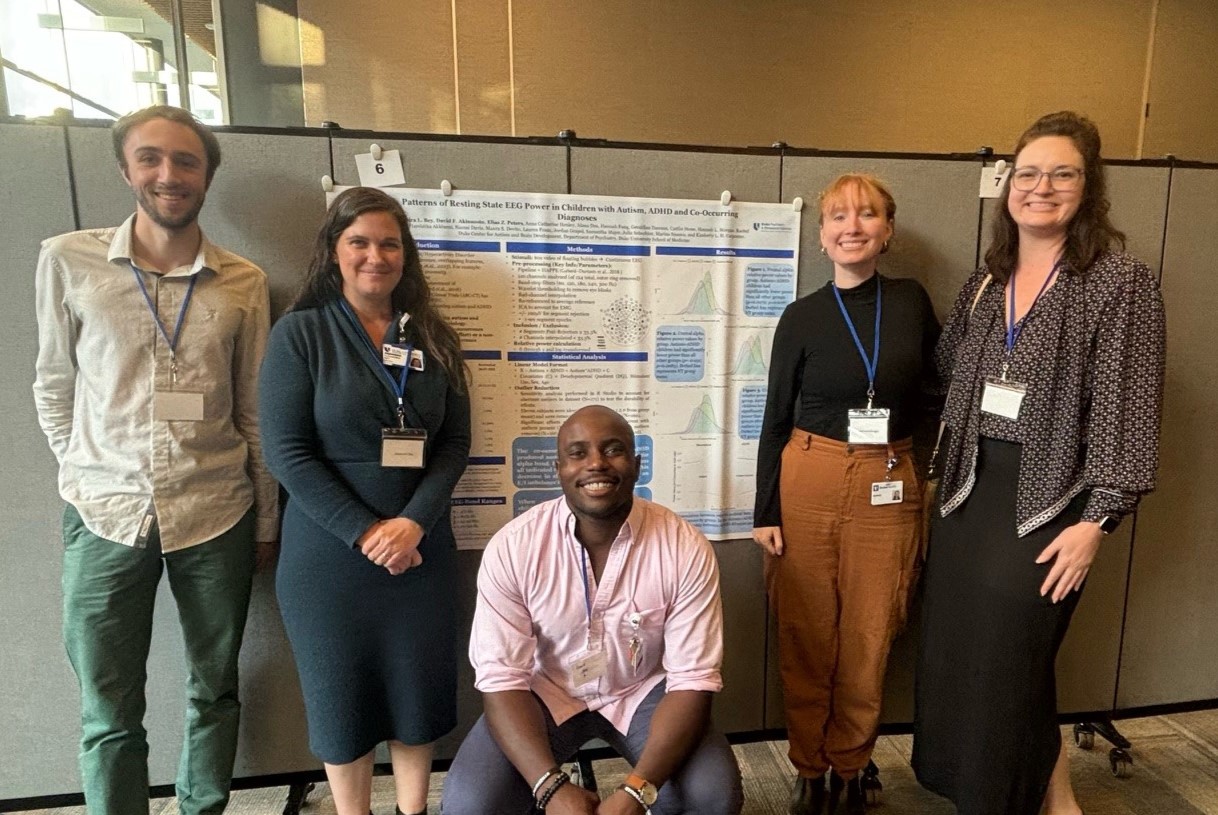
The COMET study is now in the data collection phase. The study team seeks to enroll more children, specifically those without autism, to see how their brain activity differs from autistic children.